Image generated using ChatGPT
Artificial intelligence continues to push the boundaries of what is possible. Among the latest developments is OpenAI o1, a brand-new model.
OpenAI claims that this AI can reason, thanks to its use of reinforcement learning. But why is this method so revolutionary, and how does it compare to other models like GPT-4o, Google Gemini, or Claude AI?
Reinforcement Learning: A New Approach
Unlike GPT-4o and previous models, Open AI o1 doesn’t just mimic its training patterns but uses reinforcement learning to solve problems on its own.
But what is reinforcement learning? Essentially, it’s a method where the AI learns through trial and error. It gets rewards for good actions and penalties for bad ones, which allows it to improve over time.
OpenAI commented that the training of Open AI o1 was done with a custom dataset and a new optimization algorithm. This means that the AI was trained on specific data and with advanced methods to maximize its performance. Unlike traditional models that rely on learned patterns from their training to generate responses, Open AI o1 uses reinforcement learning to autonomously solve problems.
Ending Hallucinations?
One of the main advantages of Open AI o1 is its ability to reduce AI “hallucinations.” This doesn’t mean the AI won’t make mistakes or invent answers, but OpenAI claims it’s less frequent compared to earlier versions of GPT. To clarify, hallucinations are incorrect or fabricated responses the AI sometimes generates, often due to insufficient or biased training data.
Classic models, like GPT-4o, are trained on vast amounts of text data to learn the structures and patterns of language. They typically use a neural network architecture called a “transformer,” which allows them to efficiently process long sequences of text.
These language models (LLMs—you can find out what that means here) are like students who have absorbed tons of books and try to answer questions based on what they’ve learned.
When in use, these models predict the next most likely token (word or part of a word) based on the given context. After pre-training, they can be fine-tuned for specific tasks to improve performance in certain areas.
Open AI o1 then uses a “chain of thought” to process queries, in the same way humans solve problems by going through them step by step.
What sets this new model apart from GPT-4o is its ability to solve complex problems, such as development and mathematics, much better than its predecessors while explaining its reasoning, according to OpenAI.
OpenAI even entered its new LLM into a competition called Codeforces. Programmers from around the world participate to solve complex problems, and guess what? It performed better than 89% of the human participants. For OpenAI, this is just the beginning: they explain that the next version will be capable of solving complicated problems in physics, chemistry, and biology at the level of PhD students.
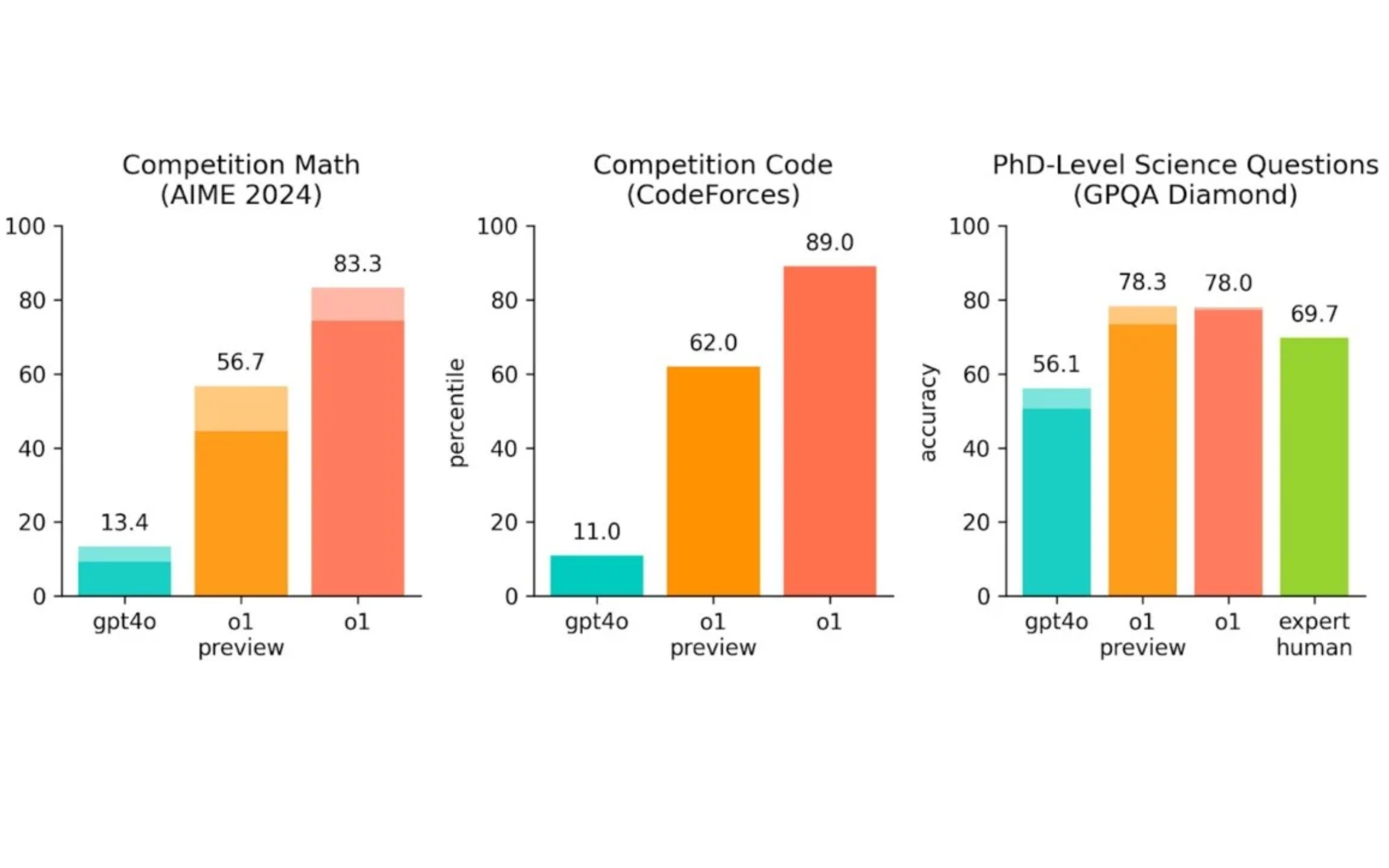
At the same time, o1 is not as powerful as GPT-4o in many areas. It is not as strong in terms of factual knowledge about the world. It also does not have the ability to browse the web or process files and images.
Obviously, we don’t always know the exact details of how each model works, as they can vary. Why? Because the companies that develop them don’t always disclose all the information about their training methods or precise architecture. This can make direct comparisons between models difficult. However, reinforcement learning seems to be a notable advancement.
How much does OpenAI GPT-o1 cost and how can it be accessed?
ChatGPT Plus and Team users have access to o1-preview and o1-mini starting today, while Enterprise and Edu users will gain access at the beginning of next week.
OpenAI announced that it plans to offer access to o1-mini to all free ChatGPT users, but has not yet set a release date.
Developer access to o1 is very expensive: in the API, o1-preview costs $15 per million input tokens, or pieces of text analyzed by the model, and $60 per million output tokens. By comparison, GPT-4o costs $5 per million input tokens and $15 per million output tokens.”